One step ahead of the burglar
A new machine learning method developed by ETH scientists enables burglary predictions even in sparsely populated areas.
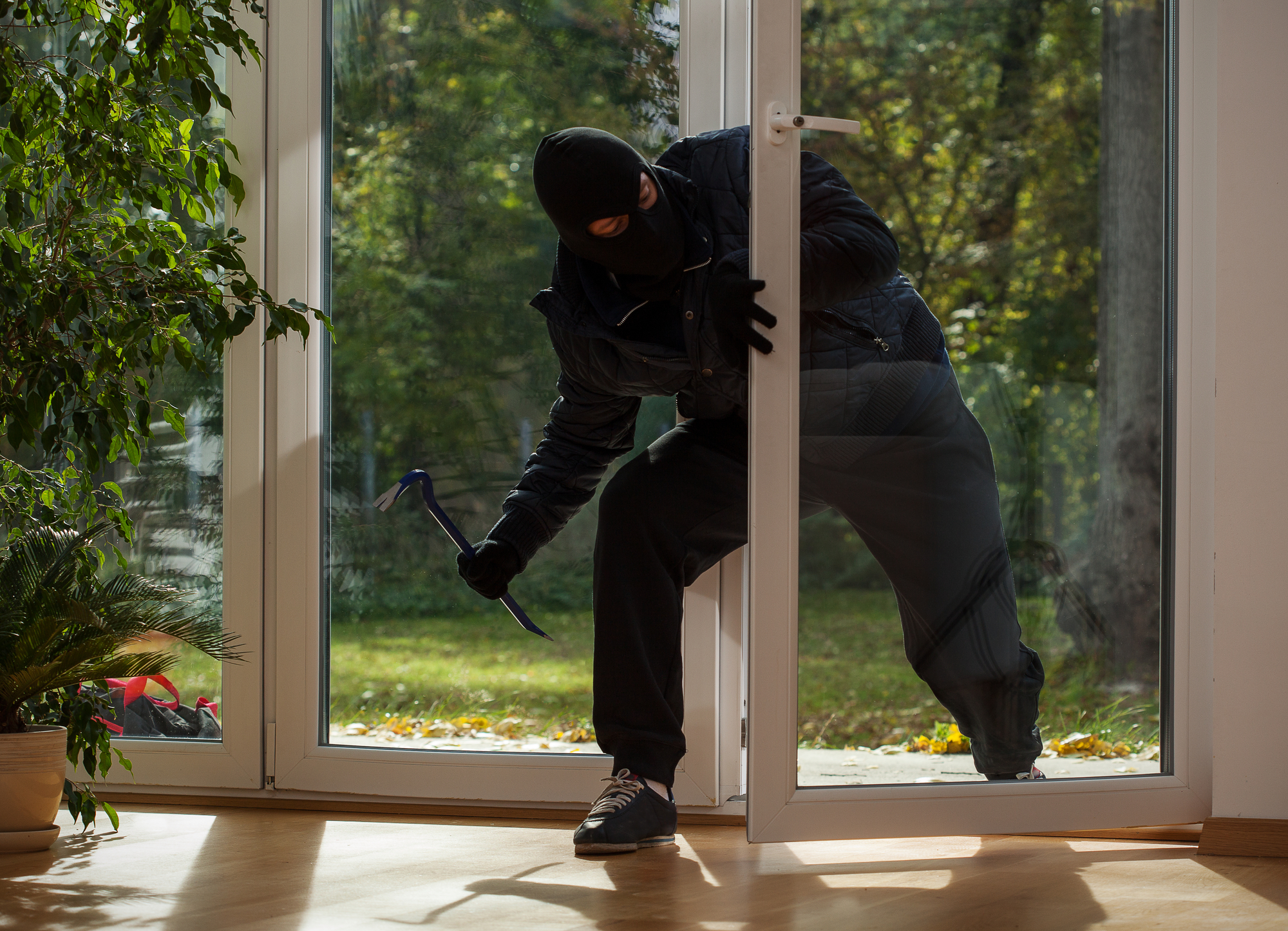
Burglaries do not occur everywhere and at all times. There are communities, neighborhoods and streets, as well as times of the year and day with lower and higher risk. From burglary statistics, machine learning can identify patterns and predict the risk of a burglary at a particular location. Computer programs can therefore help the police to identify so-called hotspots - places with a particularly high risk of burglary - for each day and deploy patrols accordingly.
Imbalance makes learning difficult
So far, however, such warning systems only work in densely populated areas, i.e. mainly in cities. That's because the computer programs need enough data to be able to recognize patterns. In rural, sparsely populated areas, criminal incidents are rarer. Statistics speak of a "class imbalance." In concrete terms, this means that for every section of road with a burglary, there are several hundred or even 1,000 without.
Algorithms work in parallel
Cristina Kadar is a computer scientist and PhD student at the Department of Management, Technology and Economics. She has developed a method that can make reliable forecasts despite unevenly distributed data. She has presented her work in the journal Decision Support Systems. Using a large data set of burglaries in the canton of Aargau, the researcher tested numerous machine learning methods, combined them and compared the hit rates. A method that uses group intelligence and combines analyses of different algorithms proved to be the most accurate.
In machine learning, an algorithm uses large data sets to train itself to classify data correctly. In the current example, it learns from variables such as time of day, location, population density and much more to classify a certain parcel of land as being at risk of burglary or not at a certain time.
The challenge was to be able to train the classification algorithms despite the small number of intrusions in the dataset. To do this, Kadar processed the dataset: Units without intrusions were randomly removed until units with intrusions and those without intrusions balanced each other. This statistical method is called "random undersampling." With this reduced data set, Kadar trained numerous classification algorithms in parallel. Their aggregated predictions yielded the intrusion forecast. Kadar used plots of 200 by 200 meters on a given day as the data unit.
While conventional warning systems primarily use burglary data, Kadar additionally fed the classification algorithms with impersonal aggregated population data, for example on population density, age structure, type of building, infrastructure (presence of schools, police stations, hospitals, roads), proximity of national borders, or temporal information such as the days of the week, holidays, daylight, and even the phase of the moon.
Hit rate better than in cities
With the new method, Kadar was able to significantly improve the hit rate compared to conventional methods. She had the computer use her method to predict where burglaries were likely to occur in the cantonal territory (hotspots). The verification showed that about 60 percent of the actual burglaries were committed in the predicted hotspots. By comparison, when hotspots were predicted using the traditional method used by police, only 53 percent of actual burglaries occurred in the predicted region. "The method achieves at least as good, and in some cases better, hit rates with unevenly distributed data than conventional methods in urban areas, where the data is denser and also more evenly distributed," Kadar says.
These findings are primarily useful for the police. The method can also be used to predict regions and times with an increased risk of burglary in less densely populated areas. However, it would also be conceivable to use the method to predict other risks: Health risks, for example, or the probability of emergency ambulance calls. The real estate industry could also use the method to forecast the price development of real estate based on spatial factors. Source: ETH News
Literature review: Kadar C., Maculan R., Feuerriegel S.: Public decision support for low population density areas: An imbalance-aware hyper-ensemble for spatio-temporal crime prediction. Decision Support Systems, 2019, doi: 10.1016/j.dss.2019.03.001
More on the topic of forecasting technology here